
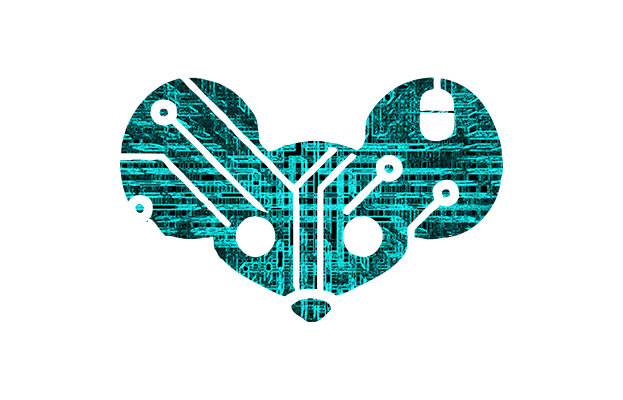
Yeah, but they encourage confining it to a virtual machine with limited access.
Yeah, but they encourage confining it to a virtual machine with limited access.
Huh. Grandpa Simpson was right. It did happen to me too.
Logic and Path-finding?
Shithole country.
Yeah, using image recognition on a screenshot of the desktop and directing a mouse around the screen with coordinates is definitely an intermediate implementation. Open Interpreter, Shell-GPT, LLM-Shell, and DemandGen make a little more sense to me for anything that can currently be done from a CLI, but I’ve never actually tested em.
I was watching users test this out and am generally impressed. At one point, Claude tried to open Firefox, but it was not responding. So it killed the process from the console and restarted. A small thing, but not something I would have expected it to overcome this early. It’s clearly not ready for prime time (by their repeated warnings), but I’m happy to see these capabilities finally making it to a foundation model’s API. It’ll be interesting to see how much remains of GUIs (or high level programming languages for that matter) if/when AI can reliably translate common language to hardware behavior.
Can I blame Trump on 9/11 or something?
Aren’t they in Macy’s now? Wait, is Macy’s still a thing?
In its latest audit of 10 leading chatbots, compiled in September, NewsGuard found that AI will repeat misinformation 18% of the time
70% of the instances where AI repeated falsehoods were in response to bad actor prompts, as opposed to leading prompts or innocent user prompts.
To be clear, it’ll be 10-30 years before AI displaces all human jobs.
an eight-year-old girl was among those killed
Calling what attention transformers do memorization is wildly inaccurate.
*Unless we’re talking about semantic memory.
It honestly blows my mind that people look at a neutral network that’s even capable of recreating short works it was trained on without having access to that text during generation… and choose to focus on IP law.
The issue is that next to the transformed output, the not-transformed input is being in use in a commercial product.
Are you only talking about the word repetition glitch?
How do you imagine those works are used?
It’s called learning, and I wish people did more of it.
This is an inaccurate understanding of what’s going on. Under the hood is a neutral network with weights and biases, not a database of copyrighted work. That neutral network was trained on a HEAVILY filtered training set (as mentioned above, 45 terabytes was reduced to 570 GB for GPT3). Getting it to bug out and generate full sections of training data from its neutral network is a fun parlor trick, but you’re not going to use it to pirate a book. People do that the old fashioned way by just adding type:pdf to their common web search.
You’ve made a lot of confident assertions without supporting them. Just like an LLM! :)
Just taking GPT 3 as an example, its training set was 45 terabytes, yes. But that set was filtered and processed down to about 570 GB. GPT 3 was only actually trained on that 570 GB. The model itself is about 700 GB. Much of the generalized intelligence of an LLM comes from abstraction to other contexts.
Table 2.2 shows the final mixture of datasets that we used in training. The CommonCrawl data was downloaded from 41 shards of monthly CommonCrawl covering 2016 to 2019, constituting 45TB of compressed plaintext before filtering and 570GB after filtering, roughly equivalent to 400 billion byte-pair-encoded tokens. Language Models are Few-Shot Learners
*Did some more looking, and that model size estimate assumes 32 bit float. It’s actually 16 bit, so the model size is 350GB… technically some compression after all!
https://www.unitree.com/g1